By Tyrone Burke
Photos by Chris Roussakis
Carleton engineering students conducting biomedical research are on the vanguard of a data-driven health-care revolution. By using Artificial Intelligence (AI), they’re mining, processing and analyzing data on an unprecedented scale.
Kevin Dick, a Biomedical Engineering PhD student, is exploring protein interactions, seeking to identify problematic bindings that lead to illness and ultimately forge a path to therapeutic treatments to prevent them. In his research, he’s using machine learning, a type of AI that allows computers to learn without being explicitly programmed.
“Ten years ago, computational approaches to protein-protein interactions were very targeted,” says Dick.
“A researcher might study a few proteins. They would model them and get high-resolution results, in that they’ll know a lot about a limited number of interactions. With machine learning, we look to do the same, but now across all possible protein interactions; we’ve truly become a big data field.”
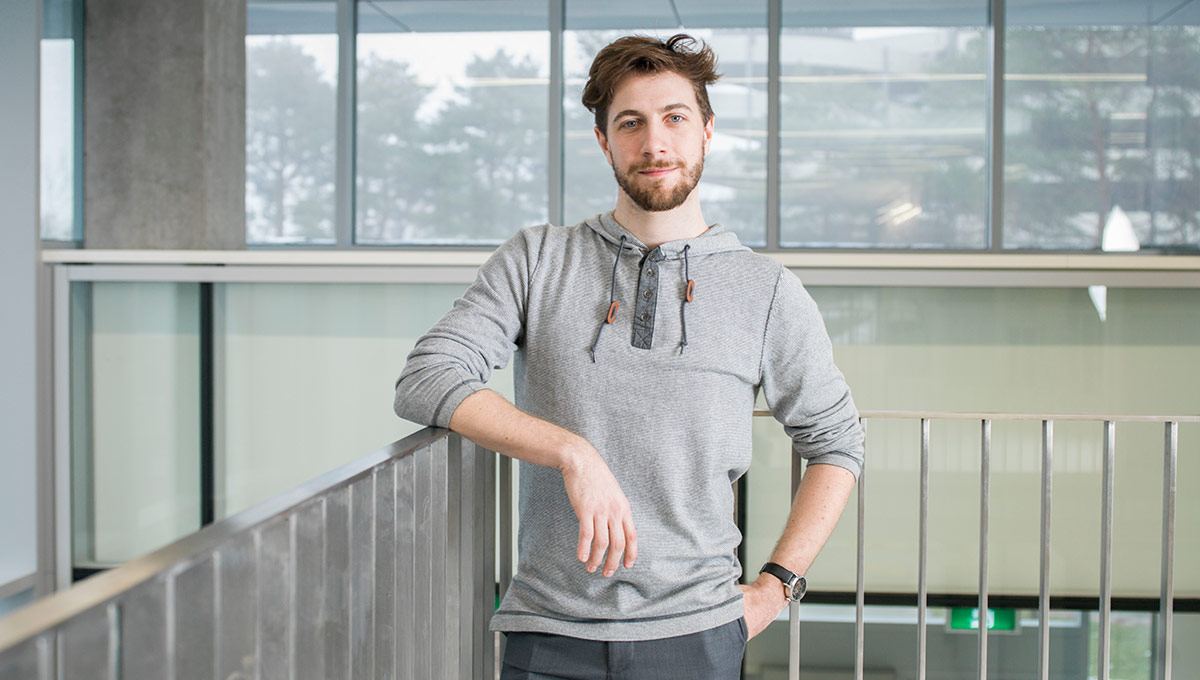
Kevin Dick
Scalable tools and algorithms can predict protein interactions in a split second. Machine learning casts a dragnet, predicting millions of them. An algorithm calculates the probability of specific types of interactions occurring between particular proteins, and produces a set of probable interactions that researchers didn’t necessarily hypothesize or anticipate.
“We’re using state-of-the-art tools,” says Dick, who uses the Carleton University Bioinformatics Research Group’s Protein-protein Interaction Prediction Engine (PIPE) and the Scoring Protein Interaction (SPRINT) algorithm developed at the University of Western Ontario.
“Very few research groups look at interaction networks of this magnitude. The potential is almost limitless. Machine learning and AI are becoming major tools to make general research more efficient.”
Helping Heart Patients
AI isn’t only transforming the lab. It’s also transforming the clinic.
Heart attacks leave a trail of destruction, and not only on families. The heart itself suffers damage and the human eye isn’t great at detecting it.
PhD candidate Fatemeh Zabihollahy uses deep learning AI to identify that damage. She receives data and imagery from Johns Hopkins University and the University of Calgary, and has developed an algorithm to process it.
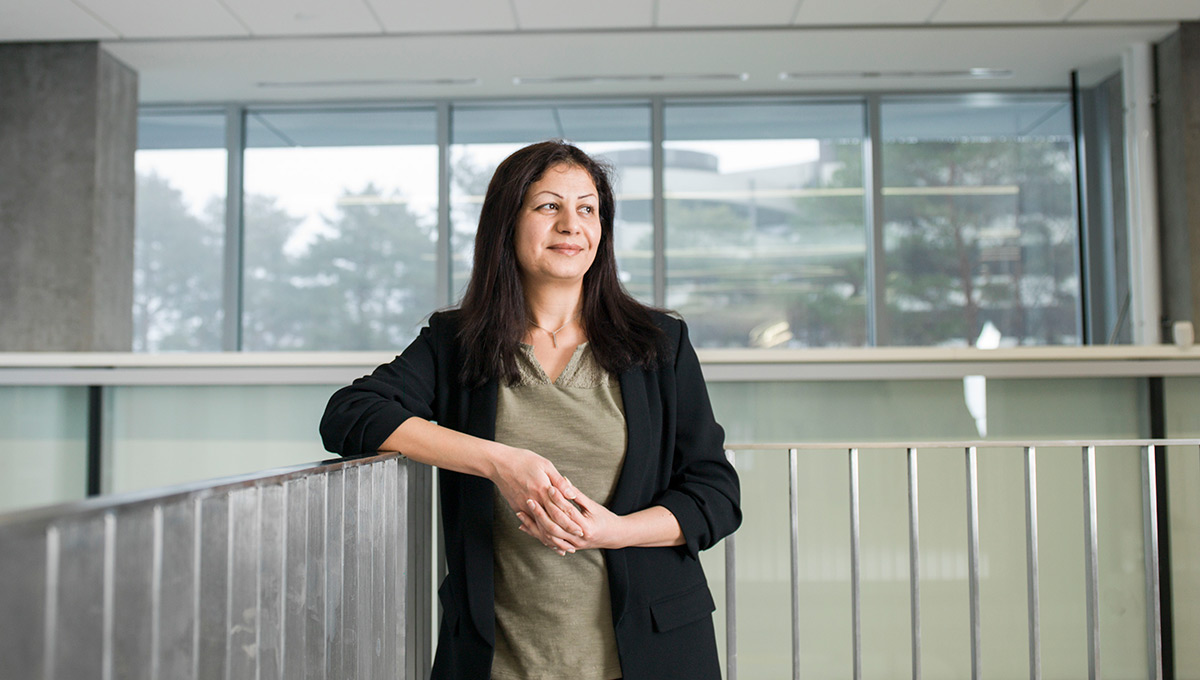
Fatemeh Zabihollahy
“Humans are good at pattern recognition and classification, but deep learning methods can help to make those tasks fully or semi-automatic, which is highly desirable for many applications, particularly medical image analysis,” explains Zabihollahy.
“Since these techniques — convolutional neural networks, recurrent neural networks – achieved some breakthrough results for certain applications like brain tumour detection, breast cancer classification, and liver and lesion segmentation, we’ve applied those techniques to cardiac Magnetic Resonance Images (MRI) and successfully distinguished between scar and healthy tissues of the heart.”
So far, results are promising. Zabihollahy’s algorithm is almost 20 per cent better at identifying damaged areas in cardiac MRI compared with conventional methods, which she says are widely employed in clinics due to their efficiency and ease of use.
Assisting Neurosurgeons
AI can also optimize delicate tasks that can only be performed by people.
Parkinson’s disease often starts with a slight hand tremor, but progresses to affect balance, speech, walking and speed of movement. The neurodegenerative condition isn’t fatal, but its complications can be.
There’s no cure, but deep brain stimulation can slow progression. PhD candidate David Lu is using machine learning to help neurosurgeons better deliver that treatment.
Deep brain stimulation implants an electric pulse generator – not unlike a pacemaker. It blocks abnormal nerve signals, mitigating Parkinson’s symptoms.
A neurosurgeon uses a Magnetic Resonance Imaging (MRI) scan to find the best location to place the electrodes. However, Lu says: “Resolution is pretty low, and when a hole is drilled into the brain, the pressure changes. Brain matter shifts, so the subthalamic nucleus or STN may not be exactly where it’s shown in MRI images.”
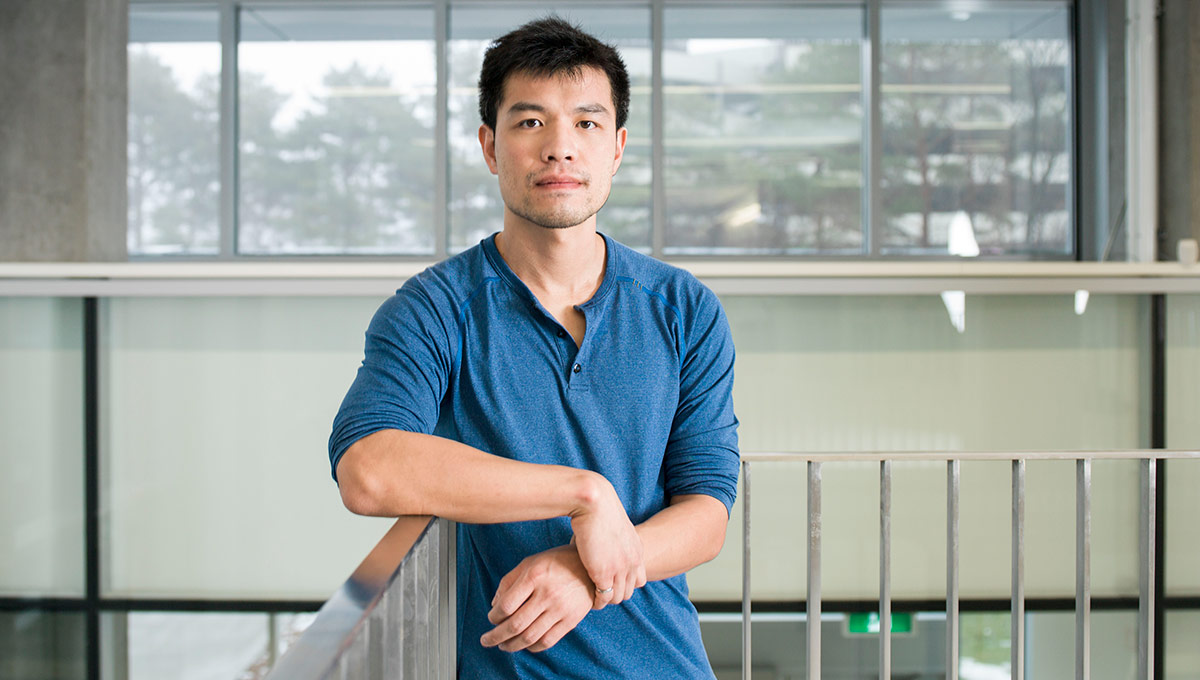
David Lu
Neurosurgeons need to re-confirm the STN’s location.
“The surgeon lowers a set of electrodes called a microelectrode recorder,” says Lu, “There’s an electrode on the tip of a needle or wire – it’s pretty long, and needs to be driven slowly along the planned trajectory. It records waves formed by neurons, which the neurosurgeon examines for the pattern STN produces.”
It often takes two to three hours to confirm the signal pattern. Lu’s research uses signal processing and machine learning to automatically label probable STN patterns.
“Machine learning recognizes a pattern pretty quickly, and algorithms can even predict a result more accurately. Google does this type of work, giving blurry animal images to people and a machine learning algorithm. Humans had probably 80 per cent success in identifying them, whereas Google’s algorithm was over 90 per cent. So it’s possible for algorithms to produce better results.”
But algorithms won’t be performing brain surgery anytime soon. Lu’s software won’t replace neurosurgeons, it will complement them.
“It’s a biomedical tool to verify their analysis,” Lu says.
“Ethics boards may not want to rely on a machine, and patients probably won’t either.”
Preventing False Alarms
Optimizing the time of professionals is a major promise of emerging biomedical engineering technologies.
Take the rapid staccato beeping of an electrocardiogram (ECG) alarm.
It’s the sound of heart failure. Except that usually, it isn’t. ECG false alarms are a health hazard and they are exceedingly common.
When doctors and nurses grow accustomed to alarms beeps, they’re more likely to ignore them. When false alarms become very frequent, workers sometimes mute them, leaving patients vulnerable. But if even one legitimate alarm is ignored, consequences can be catastrophic.
PhD candidate Mohamed Abdelazez’s research seeks to reduce false alarms and help health-care workers be more responsive in crisis.
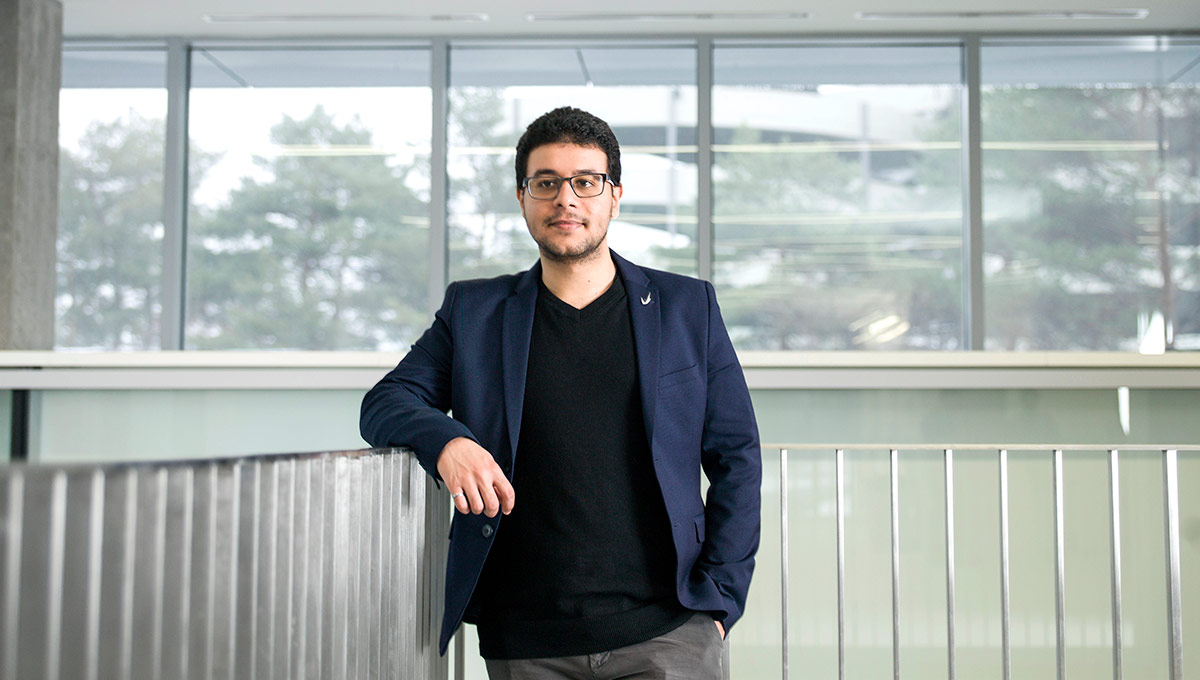
Mohamed Abdelazez
“Doctors often tell patients to move around after surgery,” Abdelazez says. “It can facilitate healing, but if they’re hooked to wires and move against them, it corrupts the signal, generating false alarms. Doctors and nurses check if they’re fine, but it’s a waste of time, and unsettling for patients.”
Abdelazez’s research makes a template of a patient’s “clean” heartbeat over a 30-second period. It analyzes all heartbeats against that beat.
“I see how much difference there is between the beat and the template — the corruption. I test over multiple thresholds. Above a certain threshold, there’s too much corruption. Below the threshold, that signal’s clean.”
An alarm with a clean signal indicates an emergency; a corrupted signal generates no alarm.
Abdelazez was drawn to the research – done with Dr. Homer Yang of the Ottawa Hospital Research Institute – by its practicality.
“Before Dr. Yang approached Carleton, he was testing myocardial ischemia monitoring systems, and he’s actually been able to save a patient. The system alerted them to an episode, a patient going into cardiac arrest. That’s the best thing ever – it might actually save someone.”
Protecting Privacy
AI in biomedical engineering can save lives, but can it preserve privacy?
Cameras are everywhere, filming everything, at all the time. And sometimes, they catch some pretty private moments.
For some, that might mean an embarrassing gaffe posted to YouTube. For the elderly, security cameras can broadcast moments like some one undressing.
Preserving dignity is one reason Zachary Baird is working on a radar-driven health monitoring system.
“It’s similar to what a security camera does,” says the engineering PhD student, “but radar keeps information confidential — you can’t tell what the person is doing and because it’s privacy-preserving, it can be used in the bathroom or the dark. It can run 24-7, mounted in the corner, and works even when people are behind objects.”
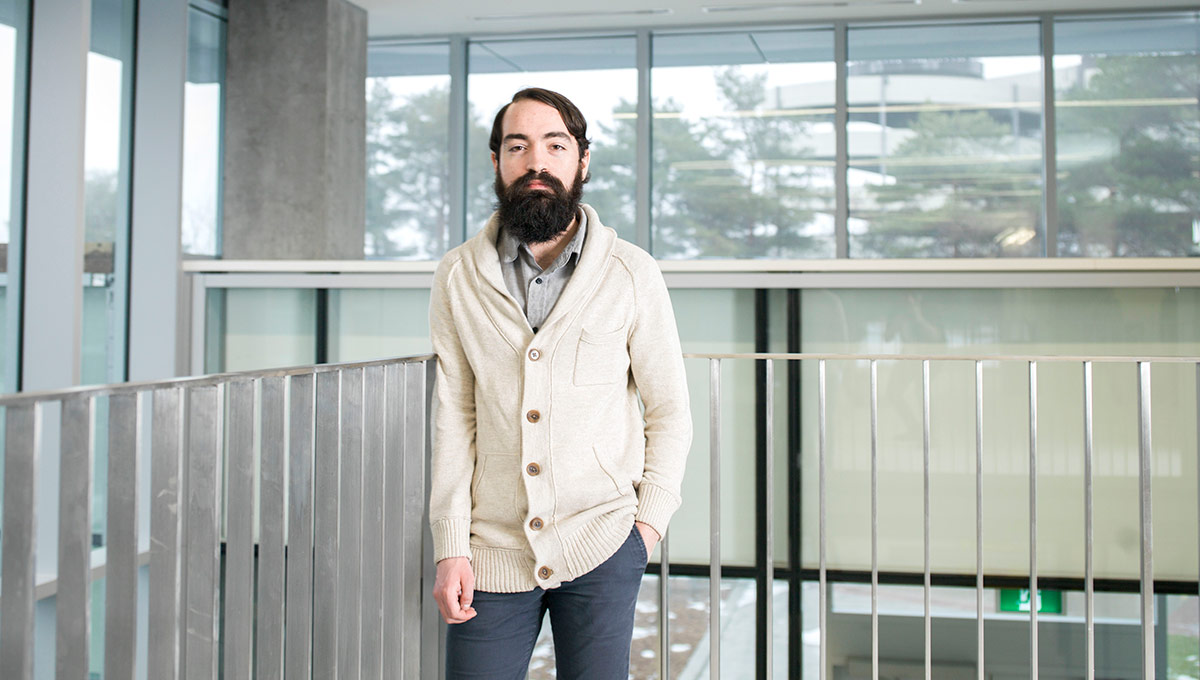
Zachary Baird
The system monitors breathing and heartbeat, and Baird is using machine learning algorithms to identify changes in balance or posture that might predict a fall. It’s aimed at seniors, but could be used by anyone in need of assisted living.
Building a system that works in all situations is challenging. Baird’s research is developing more effective ways to interpret data already being generated.
“There’s a disconnect between controlled situations and real-world context, with unknowns that need to be overcome before a robust system can give reliable results. The logic from other monitoring devices can’t be expected to work. It’s complex, non-linear signal processing.”
As the population ages and health care costs increase, hands-off monitoring systems will be necessary.
“This could alleviate the burden on family,” Baird says, “and help them know loved ones are safe. And it’s contact-free, so there’s no need to worry about them being uncomfortable.”
Abdelazez, Baird, Lu and Zabihollahy are conducting biomedical research in the Electrical and Computer Engineering PhD program. Kevin Dick is pursuing a PhD degree in Biomedical Engineering, which was launched at Carleton last fall.
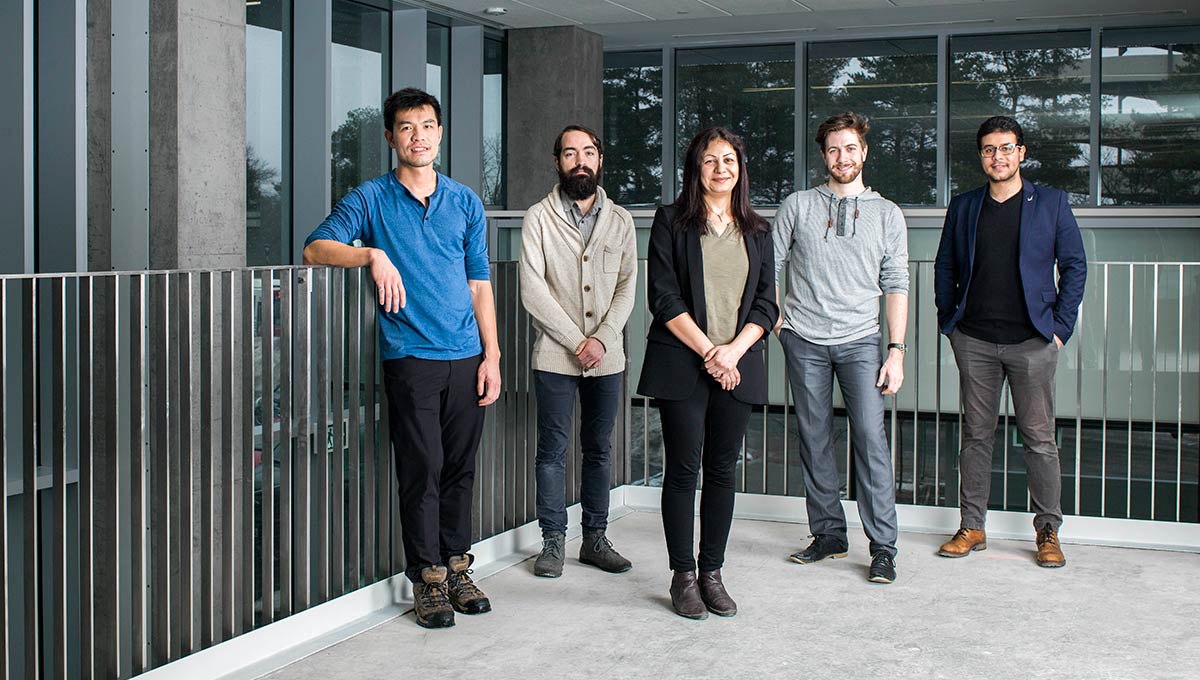
Tuesday, January 16, 2018 in Engineering, Graduate Students, Research
Share: Twitter, Facebook