By Tyrone Burke
Photos by Josh Hotz
There are more than 7.5 million kilometres of roads in North America and their conditions are constantly changing. Construction, potholes, accident scenes, new road signs and altered road geometry each present a challenge for today’s autonomous vehicle technologies, which use a combination of on-board sensors and cameras to interpret their environment.
These types of technologies are vulnerable to visual disruptions, including weather conditions. They can struggle to recognize a stop sign that’s covered in snow or ice — or even one that’s been vandalized with graffiti.
Connected vehicle technologies like Dedicated Short Range Communications (DSRC) can help resolve this issue by transmitting information about local road conditions through smart infrastructure, says Richard Yu, professor in Carleton’s School of Information Technology.
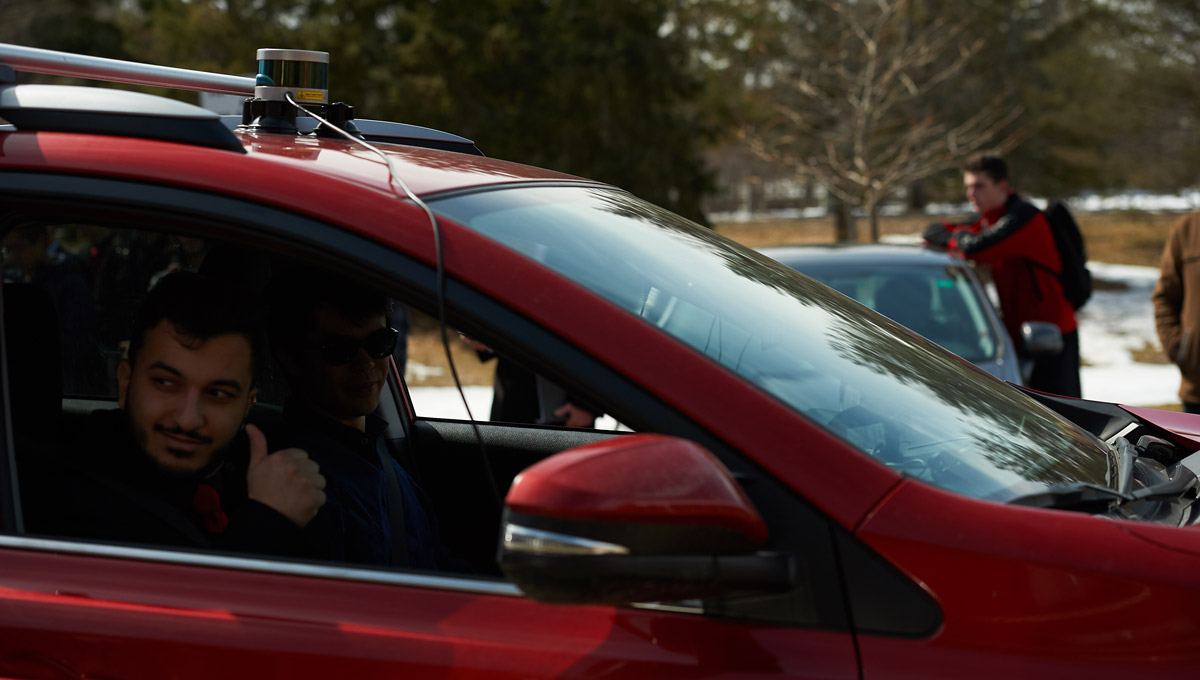
Making Autonomous Vehicles Safer
And vehicles will also need to share information from their onboard sensing equipment with each other. Called collective intelligence, this approach to data sharing could make autonomous vehicles safer by maximizing the information available to each vehicle, but transmitting so much information also introduces security vulnerabilities that Yu is working to mitigate.
His research is supported by the Canadian Safety and Security Program (CSSP), Transport Canada and Blackberry QNX and, on April 11, 2019, Yu demonstrated how they’re making collective intelligence secure.
With his team of 10 students studying information technology, systems and computer engineering and mechanical engineering, Yu modelled a malicious “spoofing attack,” in which the GPS coordinates for a stop sign on campus were altered to falsely show its location as being in the middle of the Atlantic Ocean, rather than on Library Road.
The team began by demonstrating that their Toyota RAV4 demo vehicle could stop automatically at the sign based on its actual GPS co-ordinates transmitted via DSRC. Then they broadcast the false GPS coordinates – a type of cyberattack — and their demo vehicle sped through as though there was no stop sign at all.
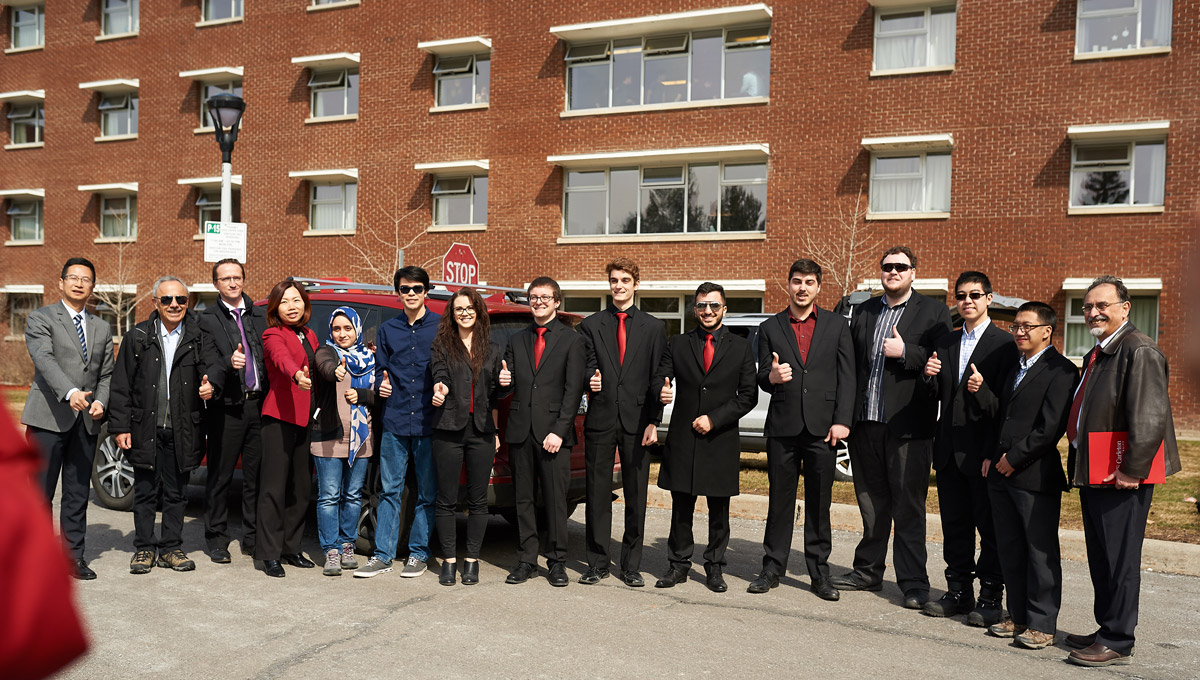
Using Machine Learning to Interpret Visual Data
Finally, the team broadcast the false message again, with the vehicle programmed to use machine learning to interpret the visual data from its front-facing camera, as well as process the GPS coordinates. This enabled the vehicle to recognize that it was approaching a stop sign and stop – even though it had been provided with false data about that stop sign’s location.
For autonomous vehicles to attain necessary levels of safety, they will need to have redundant systems like these. Every type of technology has its own set of strengths, weaknesses and vulnerabilities to attack. To safely navigate crowded streets and difficult weather conditions, autonomous vehicles will need to simultaneously process data generated by multiple types of sensors and data transmissions.
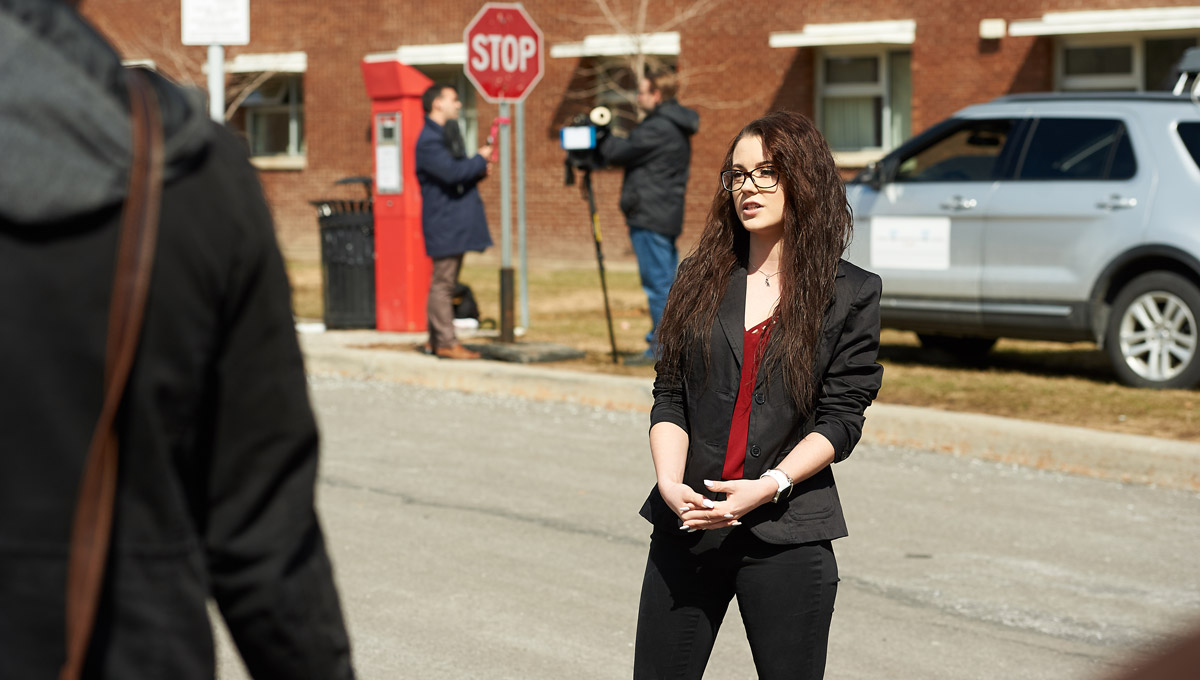
Kaitlyn Harris
“The biggest challenge was interfacing these two technologies,” says Kaitlyn Harris, a fourth-year student who contributed to preparing the RAV4 for the demonstration.
“It was something that had never been done before — the two systems were completely separate. Now that the two systems are able to communicate. This can be expanded from stop signs to situations like four-way stops, traffic lights, crosswalks or railways.”
Wednesday, April 17, 2019 in Faculty of Engineering and Design
Share: Twitter, Facebook