By Ty Burke
Photos by Melanie Mathieu
Everybody eats. So, the integrity of our food supply affects us all, but maintaining it is no easy task.
Every year, there are well over a hundred food safety recalls in Canada. When pathogens such as E. coli and salmonella are detected, we take products off the shelves. But that doesn’t always happen quickly enough. In some cases, we only learn that food is contaminated after people start getting sick.
And then there’s food fraud, which is even more difficult to detect. This happens when ingredients are replaced with cheaper substitutes — like maple syrup cut with cheaper corn syrup. This often doesn’t cause illness, but it does cost consumers money, because they don’t actually get what they’re paying for.
Carleton University chemistry researcher Yaxi Hu’s Food Analytical Chemistry and Technology (FACT) Lab is helping us understand what we’re actually eating. She is developing portable, easy-to-use testing techniques for assessing food safety, quality and authenticity.
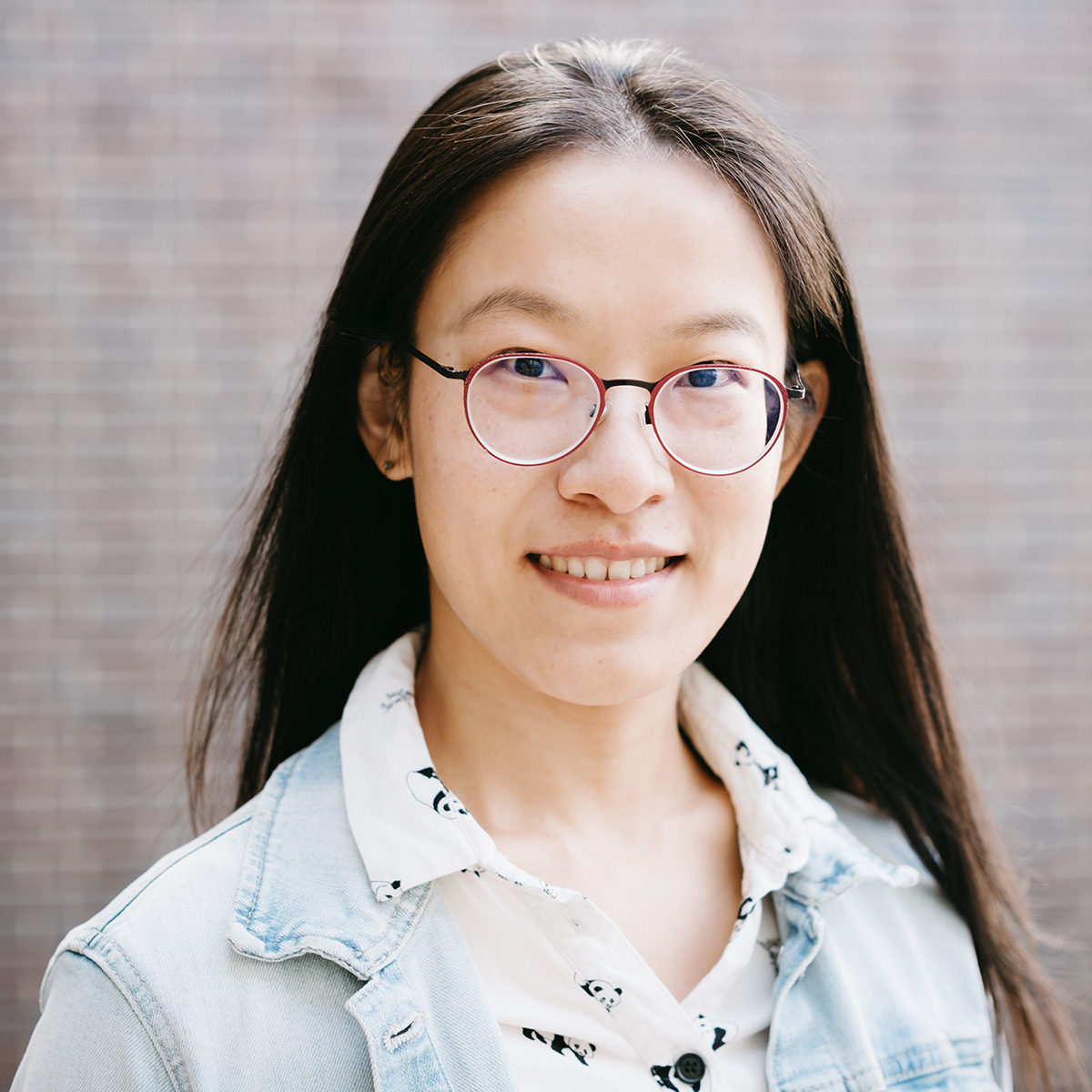
Carleton University chemistry researcher Yaxi Hu
“This is a worldwide problem,” says Hu.
“Studies have shown that in fish, the mislabelling rate can be as high as 30 per cent.”
Microfluidic devices are one technology that could make it easier to identify mislabelling. These are something like a rapid test for COVID-19 and detect whether a specific type of genetic material is present on a small test strip.
“If pomegranate juice has been adulterated with apple juice, it will have two different DNA sequences,” says Hu.
“If you can analyze these, you can determine if apple juice is present. Conventional methods like a PCR test require specific instruments and take a few hours to get results. But with this device, analysis can be completed in one hour, and doesn’t require expensive instruments. The DNA is extracted and amplified on the paper-based microfluidic device, and we can directly see the results by the device’s colour change.”
One advantage of this approach is that it can be implemented in locations without access to lab equipment. And microfluidic devices can also be used to detect the genetic signatures of dangerous pathogens like salmonella and campylobacter, both common bacterial causes of human gastroenteritis.
But while they can be adapted to detect different substances, microfluidic devices are an ultimately targeted method that detect only one signature at a time. A device designed to detect pomegranate DNA won’t tell you whether salmonella is present, and vice versa. And that speaks to one of the major challenges of food authentication — you don’t always know what food will be adulterated with.
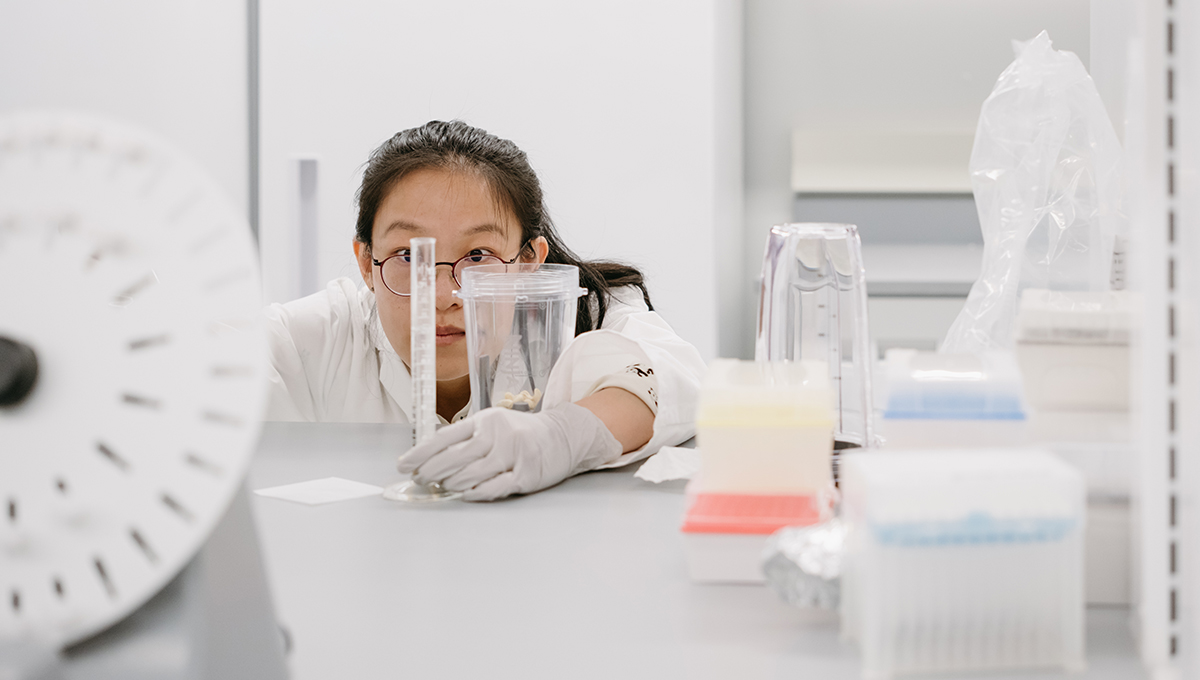
Untargeted Food Fraud Detection with Machine Learning
In 2008, about 300,000 infants in China got sick from contaminated milk, which had been intentionally adulterated with a chemical called melamine. This boosts milk’s apparent protein content and allows producers to get a higher price for their product. But melamine causes crystals to form in the human body, and while adults are able to process them, infants cannot. This can cause kidney failure, and even lead to death. But even a test designed to detect melamine wouldn’t necessarily prevent something like this from happening again.
“We could develop a method that targets melamine. But if next time they use some other compound, that method will fail,” says Hu.
“So that instead of doing this, we are trying to develop untargeted methods that can differentiate authentic products from adulterated ones.”
Every chemical has a unique composition and spectral signature — something like a human fingerprint. Hu is developing spectroscopy-based methods to capture that signature and match it with a reference library of known chemicals or food products. One technique uses vibrational spectroscopy. This excites a sample by shooting it with infrared laser, and that generates signals that are captured by a sensor, which records how the sample absorbs or scatters photons.
That is the sample’s spectral signature, and it can be matched to the spectral library to determine what is present — even if you don’t know specifically what you’re looking for. But the matching is a data-intensive process that’s best left to computers.
“Machine learning can be used to classify or even quantify chemicals,” Hu says.
“When you do not know exactly what you are looking for, a classification and quantification model can tell you what is in a sample and gives you instant results. So, you will know if a product is authentic or not.”
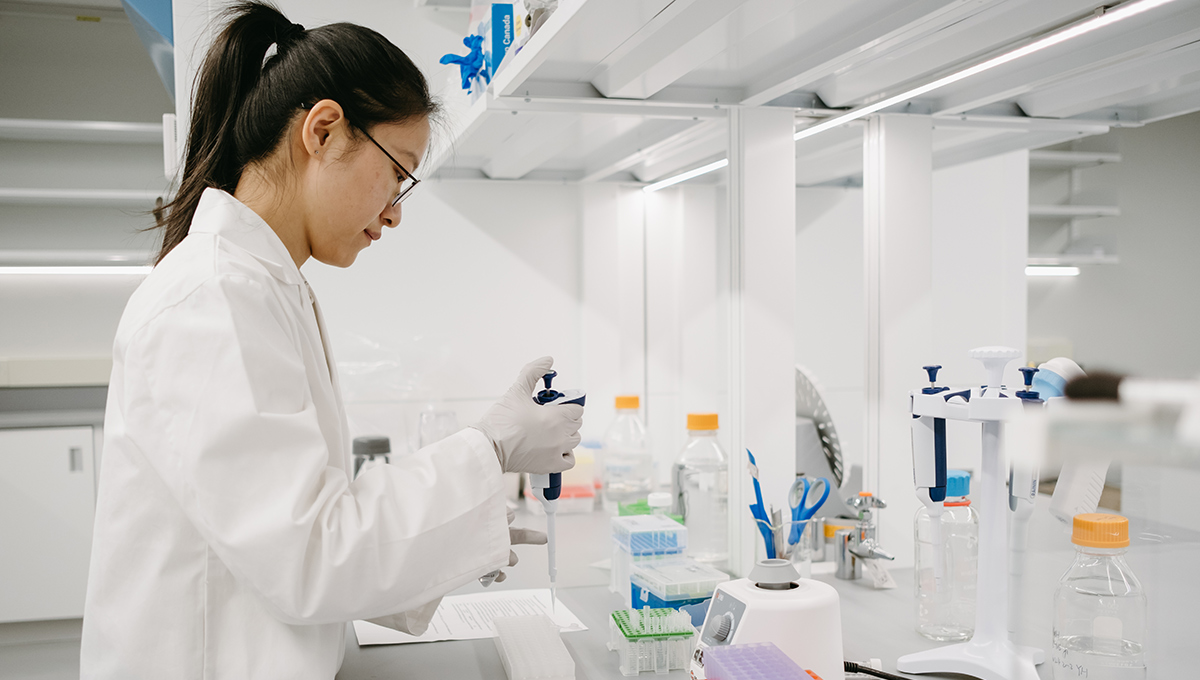